mirror of
https://github.com/babysor/MockingBird.git
synced 2024-03-22 13:11:31 +08:00
164 lines
8.7 KiB
Markdown
164 lines
8.7 KiB
Markdown
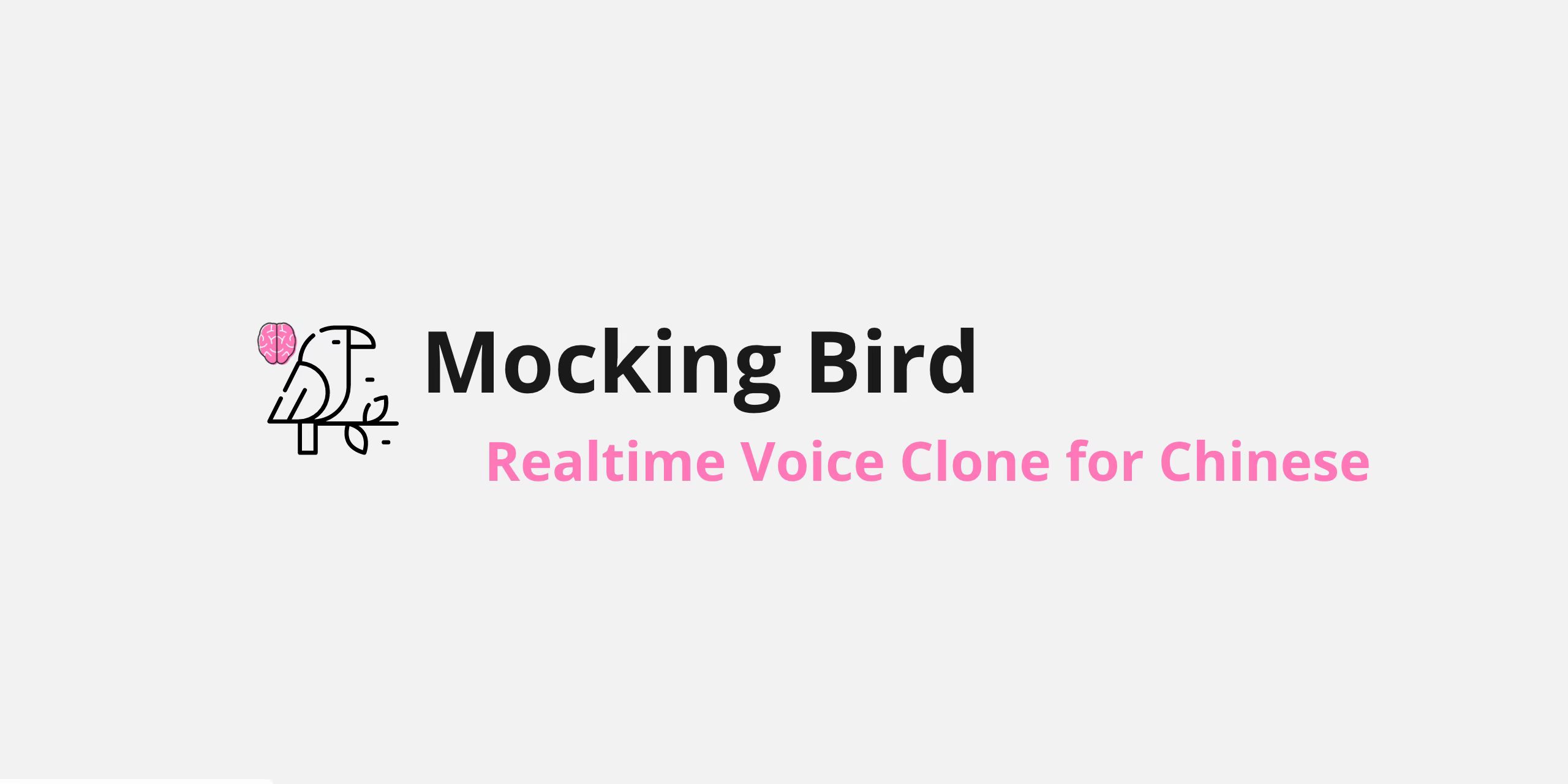
|
||
|
||
|
||
[](http://choosealicense.com/licenses/mit/)
|
||
|
||
> English | [中文](README-CN.md)
|
||
|
||
## Features
|
||
🌍 **Chinese** supported mandarin and tested with multiple datasets: aidatatang_200zh, magicdata, aishell3, and etc.
|
||
|
||
🤩 **PyTorch** worked for pytorch, tested in version of 1.9.0(latest in August 2021), with GPU Tesla T4 and GTX 2060
|
||
|
||
🌍 **Windows + Linux** run in both Windows OS and linux OS (even in M1 MACOS)
|
||
|
||
🤩 **Easy & Awesome** effect with only newly-trained synthesizer, by reusing the pretrained encoder/vocoder
|
||
|
||
🌍 **Webserver Ready** to serve your result with remote calling
|
||
|
||
### [DEMO VIDEO](https://www.bilibili.com/video/BV17Q4y1B7mY/)
|
||
|
||
## Quick Start
|
||
|
||
### 1. Install Requirements
|
||
> Follow the original repo to test if you got all environment ready.
|
||
**Python 3.7 or higher ** is needed to run the toolbox.
|
||
|
||
* Install [PyTorch](https://pytorch.org/get-started/locally/).
|
||
> If you get an `ERROR: Could not find a version that satisfies the requirement torch==1.9.0+cu102 (from versions: 0.1.2, 0.1.2.post1, 0.1.2.post2 )` This error is probably due to a low version of python, try using 3.9 and it will install successfully
|
||
* Install [ffmpeg](https://ffmpeg.org/download.html#get-packages).
|
||
* Run `pip install -r requirements.txt` to install the remaining necessary packages.
|
||
* Install webrtcvad `pip install webrtcvad-wheels`(If you need)
|
||
> Note that we are using the pretrained encoder/vocoder but synthesizer, since the original model is incompatible with the Chinese sympols. It means the demo_cli is not working at this moment.
|
||
### 2. Prepare your models
|
||
You can either train your models or use existing ones:
|
||
#### 2.1. Train synthesizer with your dataset
|
||
* Download dataset and unzip: make sure you can access all .wav in folder
|
||
* Preprocess with the audios and the mel spectrograms:
|
||
`python pre.py <datasets_root>`
|
||
Allowing parameter `--dataset {dataset}` to support aidatatang_200zh, magicdata, aishell3, etc.
|
||
|
||
* Train the synthesizer:
|
||
`python synthesizer_train.py mandarin <datasets_root>/SV2TTS/synthesizer`
|
||
|
||
* Go to next step when you see attention line show and loss meet your need in training folder *synthesizer/saved_models/*.
|
||
|
||
#### 2.2 Use pretrained model of synthesizer
|
||
> Thanks to the community, some models will be shared:
|
||
|
||
| author | Download link | Preview Video | Info |
|
||
| --- | ----------- | ----- |----- |
|
||
| @myself | https://pan.baidu.com/s/1VHSKIbxXQejtxi2at9IrpA [Baidu](https://pan.baidu.com/s/1VHSKIbxXQejtxi2at9IrpA ) code:i183 | | 200k steps only trained by aidatatang_200zh
|
||
|@FawenYo | https://drive.google.com/file/d/1H-YGOUHpmqKxJ9FRc6vAjPuqQki24UbC/view?usp=sharing [Baidu Pan](https://pan.baidu.com/s/1vSYXO4wsLyjnF3Unl-Xoxg) Code:1024 | [input](https://github.com/babysor/MockingBird/wiki/audio/self_test.mp3) [output](https://github.com/babysor/MockingBird/wiki/audio/export.wav) | 200k steps with local accent of Taiwan
|
||
|@miven| https://pan.baidu.com/s/1PI-hM3sn5wbeChRryX-RCQ code:2021 | https://www.bilibili.com/video/BV1uh411B7AD/
|
||
|
||
#### 2.3 Train vocoder (Optional)
|
||
> note: vocoder has little difference in effect, so you may not need to train a new one.
|
||
* Preprocess the data:
|
||
`python vocoder_preprocess.py <datasets_root> -m <synthesizer_model_path>`
|
||
> `<datasets_root>` replace with your dataset root,`<synthesizer_model_path>`replace with directory of your best trained models of sythensizer, e.g. *sythensizer\saved_mode\xxx*
|
||
|
||
* Train the wavernn vocoder:
|
||
`python vocoder_train.py mandarin <datasets_root>`
|
||
|
||
* Train the hifigan vocoder
|
||
`python vocoder_train.py mandarin <datasets_root> hifigan`
|
||
|
||
### 3. Launch
|
||
#### 3.1 Using the web server
|
||
You can then try to run:`python web.py` and open it in browser, default as `http://localhost:8080`
|
||
|
||
#### 3.2 Using the Toolbox
|
||
You can then try the toolbox:
|
||
`python demo_toolbox.py -d <datasets_root>`
|
||
|
||
## Reference
|
||
> This repository is forked from [Real-Time-Voice-Cloning](https://github.com/CorentinJ/Real-Time-Voice-Cloning) which only support English.
|
||
|
||
| URL | Designation | Title | Implementation source |
|
||
| --- | ----------- | ----- | --------------------- |
|
||
| [2010.05646](https://arxiv.org/abs/2010.05646) | HiFi-GAN (vocoder)| Generative Adversarial Networks for Efficient and High Fidelity Speech Synthesis | This repo |
|
||
|[**1806.04558**](https://arxiv.org/pdf/1806.04558.pdf) | **SV2TTS** | **Transfer Learning from Speaker Verification to Multispeaker Text-To-Speech Synthesis** | This repo |
|
||
|[1802.08435](https://arxiv.org/pdf/1802.08435.pdf) | WaveRNN (vocoder) | Efficient Neural Audio Synthesis | [fatchord/WaveRNN](https://github.com/fatchord/WaveRNN) |
|
||
|[1703.10135](https://arxiv.org/pdf/1703.10135.pdf) | Tacotron (synthesizer) | Tacotron: Towards End-to-End Speech Synthesis | [fatchord/WaveRNN](https://github.com/fatchord/WaveRNN)
|
||
|[1710.10467](https://arxiv.org/pdf/1710.10467.pdf) | GE2E (encoder)| Generalized End-To-End Loss for Speaker Verification | This repo |
|
||
|
||
## F Q&A
|
||
#### 1.Where can I download the dataset?
|
||
| Dataset | Original Source | Alternative Sources |
|
||
| --- | ----------- | ---------------|
|
||
| aidatatang_200zh | [OpenSLR](http://www.openslr.org/62/) | [Google Drive](https://drive.google.com/file/d/110A11KZoVe7vy6kXlLb6zVPLb_J91I_t/view?usp=sharing) |
|
||
| magicdata | [OpenSLR](http://www.openslr.org/68/) | [Google Drive (Dev set)](https://drive.google.com/file/d/1g5bWRUSNH68ycC6eNvtwh07nX3QhOOlo/view?usp=sharing) |
|
||
| aishell3 | [OpenSLR](https://www.openslr.org/93/) | [Google Drive](https://drive.google.com/file/d/1shYp_o4Z0X0cZSKQDtFirct2luFUwKzZ/view?usp=sharing) |
|
||
> After unzip aidatatang_200zh, you need to unzip all the files under `aidatatang_200zh\corpus\train`
|
||
|
||
#### 2.What is`<datasets_root>`?
|
||
If the dataset path is `D:\data\aidatatang_200zh`,then `<datasets_root>` is`D:\data`
|
||
|
||
#### 3.Not enough VRAM
|
||
Train the synthesizer:adjust the batch_size in `synthesizer/hparams.py`
|
||
```
|
||
//Before
|
||
tts_schedule = [(2, 1e-3, 20_000, 12), # Progressive training schedule
|
||
(2, 5e-4, 40_000, 12), # (r, lr, step, batch_size)
|
||
(2, 2e-4, 80_000, 12), #
|
||
(2, 1e-4, 160_000, 12), # r = reduction factor (# of mel frames
|
||
(2, 3e-5, 320_000, 12), # synthesized for each decoder iteration)
|
||
(2, 1e-5, 640_000, 12)], # lr = learning rate
|
||
//After
|
||
tts_schedule = [(2, 1e-3, 20_000, 8), # Progressive training schedule
|
||
(2, 5e-4, 40_000, 8), # (r, lr, step, batch_size)
|
||
(2, 2e-4, 80_000, 8), #
|
||
(2, 1e-4, 160_000, 8), # r = reduction factor (# of mel frames
|
||
(2, 3e-5, 320_000, 8), # synthesized for each decoder iteration)
|
||
(2, 1e-5, 640_000, 8)], # lr = learning rate
|
||
```
|
||
|
||
Train Vocoder-Preprocess the data:adjust the batch_size in `synthesizer/hparams.py`
|
||
```
|
||
//Before
|
||
### Data Preprocessing
|
||
max_mel_frames = 900,
|
||
rescale = True,
|
||
rescaling_max = 0.9,
|
||
synthesis_batch_size = 16, # For vocoder preprocessing and inference.
|
||
//After
|
||
### Data Preprocessing
|
||
max_mel_frames = 900,
|
||
rescale = True,
|
||
rescaling_max = 0.9,
|
||
synthesis_batch_size = 8, # For vocoder preprocessing and inference.
|
||
```
|
||
|
||
Train Vocoder-Train the vocoder:adjust the batch_size in `vocoder/wavernn/hparams.py`
|
||
```
|
||
//Before
|
||
# Training
|
||
voc_batch_size = 100
|
||
voc_lr = 1e-4
|
||
voc_gen_at_checkpoint = 5
|
||
voc_pad = 2
|
||
|
||
//After
|
||
# Training
|
||
voc_batch_size = 6
|
||
voc_lr = 1e-4
|
||
voc_gen_at_checkpoint = 5
|
||
voc_pad =2
|
||
```
|
||
|
||
#### 4.If it happens `RuntimeError: Error(s) in loading state_dict for Tacotron: size mismatch for encoder.embedding.weight: copying a param with shape torch.Size([70, 512]) from checkpoint, the shape in current model is torch.Size([75, 512]).`
|
||
Please refer to issue [#37](https://github.com/babysor/MockingBird/issues/37)
|
||
|
||
#### 5. How to improve CPU and GPU occupancy rate?
|
||
Adjust the batch_size as appropriate to improve
|
||
|
||
|
||
#### 6. What if it happens `the page file is too small to complete the operation`
|
||
Please refer to this [video](https://www.youtube.com/watch?v=Oh6dga-Oy10&ab_channel=CodeProf) and change the virtual memory to 100G (102400), for example : When the file is placed in the D disk, the virtual memory of the D disk is changed.
|
||
|
||
#### 7. When should I stop during training?
|
||
FYI, my attention came after 18k steps and loss became lower than 0.4 after 50k steps.
|
||
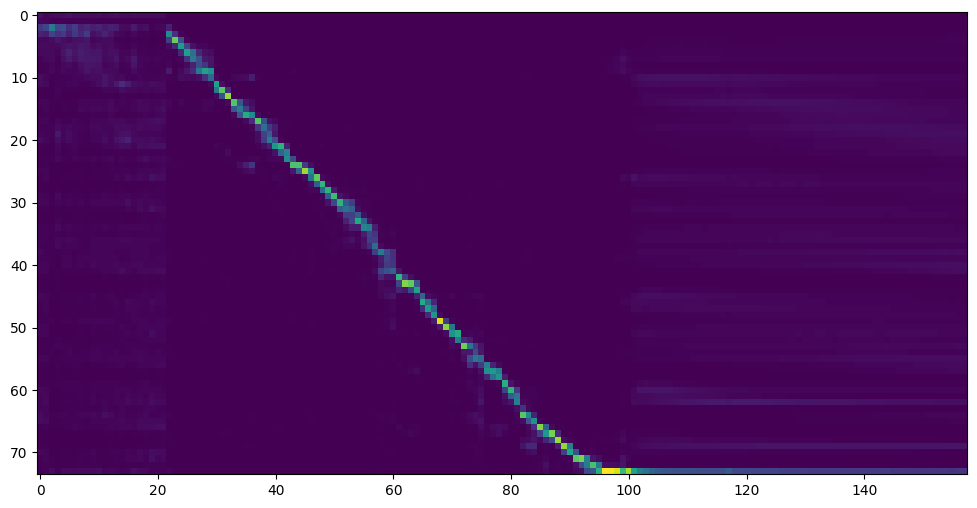
|
||
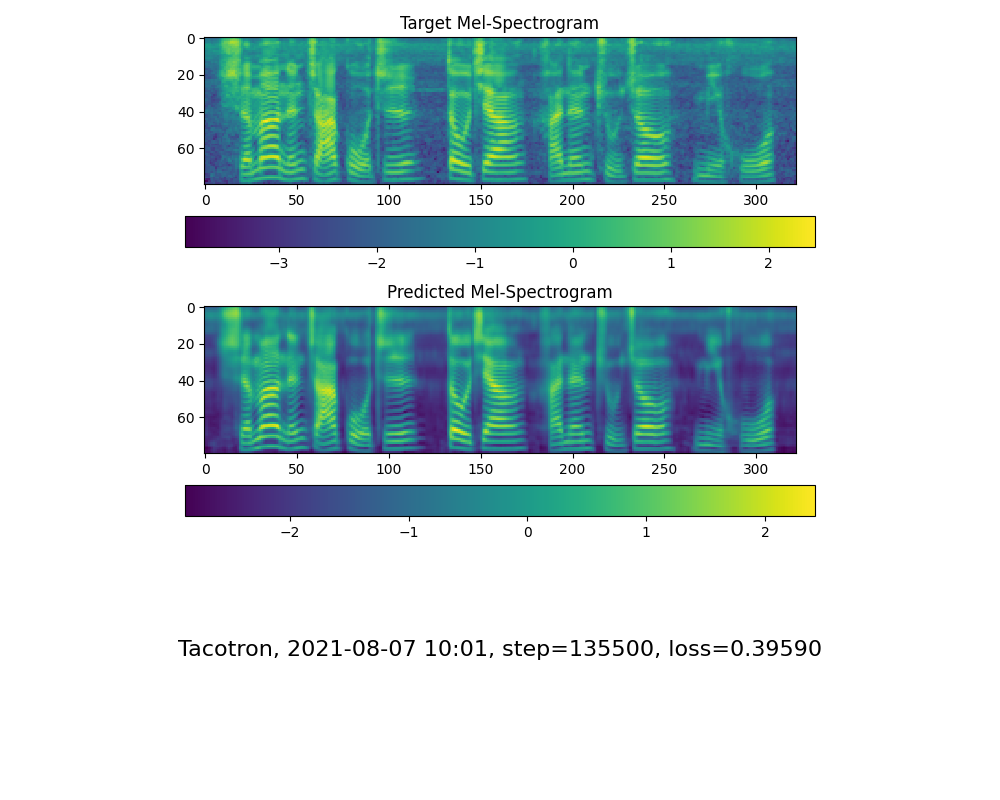
|