224 lines
8.8 KiB
Markdown
224 lines
8.8 KiB
Markdown
## 实时语音克隆 - 中文/普通话
|
||
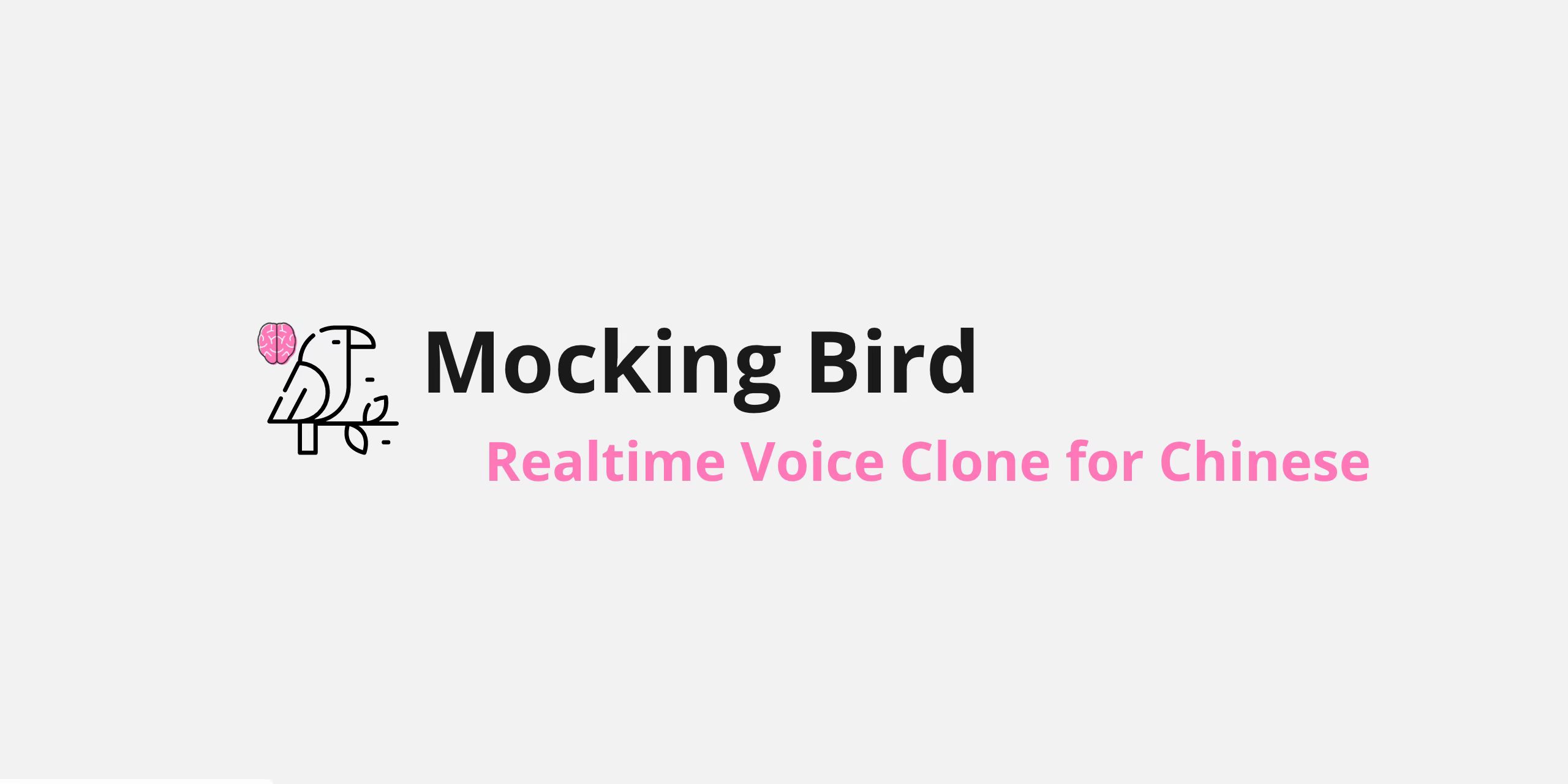
|
||
|
||
[](http://choosealicense.com/licenses/mit/)
|
||
|
||
### [English](README.md) | 中文
|
||
|
||
### [DEMO VIDEO](https://www.bilibili.com/video/BV17Q4y1B7mY/) | [Wiki教程](https://github.com/babysor/MockingBird/wiki/Quick-Start-(Newbie)) | [训练教程](https://vaj2fgg8yn.feishu.cn/docs/doccn7kAbr3SJz0KM0SIDJ0Xnhd)
|
||
|
||
## 特性
|
||
🌍 **中文** 支持普通话并使用多种中文数据集进行测试:aidatatang_200zh, magicdata, aishell3, biaobei, MozillaCommonVoice, data_aishell 等
|
||
|
||
🤩 **Easy & Awesome** 仅需下载或新训练合成器(synthesizer)就有良好效果,复用预训练的编码器/声码器,或实时的HiFi-GAN作为vocoder
|
||
|
||
🌍 **Webserver Ready** 可伺服你的训练结果,供远程调用。
|
||
|
||
🤩 **感谢各位小伙伴的支持,本项目将开启新一轮的更新**
|
||
|
||
## 1.快速开始
|
||
### 1.1 建议环境
|
||
- Ubuntu 18.04
|
||
- Cuda 11.7 && CuDNN 8.5.0
|
||
- Python 3.8 或 3.9
|
||
- Pytorch 2.0.1 <post cuda-11.7>
|
||
### 1.2 环境配置
|
||
```shell
|
||
# 下载前建议更换国内镜像源
|
||
|
||
conda create -n sound python=3.9
|
||
|
||
conda activate sound
|
||
|
||
git clone https://github.com/babysor/MockingBird.git
|
||
|
||
cd MockingBird
|
||
|
||
pip install -r requirements.txt
|
||
|
||
pip install webrtcvad-wheels
|
||
|
||
pip install torch torchvision torchaudio --index-url https://download.pytorch.org/whl/cu117
|
||
```
|
||
|
||
### 1.3 模型准备
|
||
> 当实在没有设备或者不想慢慢调试,可以使用社区贡献的模型(欢迎持续分享):
|
||
|
||
| 作者 | 下载链接 | 效果预览 | 信息 |
|
||
| --- | ----------- | ----- | ----- |
|
||
| 作者 | https://pan.baidu.com/s/1iONvRxmkI-t1nHqxKytY3g [百度盘链接](https://pan.baidu.com/s/1iONvRxmkI-t1nHqxKytY3g) 4j5d | | 75k steps 用3个开源数据集混合训练
|
||
| 作者 | https://pan.baidu.com/s/1fMh9IlgKJlL2PIiRTYDUvw [百度盘链接](https://pan.baidu.com/s/1fMh9IlgKJlL2PIiRTYDUvw) 提取码:om7f | | 25k steps 用3个开源数据集混合训练, 切换到tag v0.0.1使用
|
||
|@FawenYo | https://drive.google.com/file/d/1H-YGOUHpmqKxJ9FRc6vAjPuqQki24UbC/view?usp=sharing [百度盘链接](https://pan.baidu.com/s/1vSYXO4wsLyjnF3Unl-Xoxg) 提取码:1024 | [input](https://github.com/babysor/MockingBird/wiki/audio/self_test.mp3) [output](https://github.com/babysor/MockingBird/wiki/audio/export.wav) | 200k steps 台湾口音需切换到tag v0.0.1使用
|
||
|@miven| https://pan.baidu.com/s/1PI-hM3sn5wbeChRryX-RCQ 提取码:2021 | https://www.bilibili.com/video/BV1uh411B7AD/ | 150k steps 注意:根据[issue](https://github.com/babysor/MockingBird/issues/37)修复 并切换到tag v0.0.1使用
|
||
|
||
### 1.4 文件结构准备
|
||
文件结构准备如下所示,算法将自动遍历synthesizer下的.pt模型文件。
|
||
```
|
||
# 以第一个 pretrained-11-7-21_75k.pt 为例
|
||
|
||
└── data
|
||
└── ckpt
|
||
└── synthesizer
|
||
└── pretrained-11-7-21_75k.pt
|
||
```
|
||
### 1.5 运行
|
||
```
|
||
python web.py
|
||
```
|
||
|
||
## 2.模型训练
|
||
### 2.1 数据准备
|
||
#### 2.1.1 数据下载
|
||
``` shell
|
||
# aidatatang_200zh
|
||
|
||
wget https://openslr.elda.org/resources/62/aidatatang_200zh.tgz
|
||
```
|
||
``` shell
|
||
# MAGICDATA
|
||
|
||
wget https://openslr.magicdatatech.com/resources/68/train_set.tar.gz
|
||
|
||
wget https://openslr.magicdatatech.com/resources/68/dev_set.tar.gz
|
||
|
||
wget https://openslr.magicdatatech.com/resources/68/test_set.tar.gz
|
||
```
|
||
``` shell
|
||
# AISHELL-3
|
||
|
||
wget https://openslr.elda.org/resources/93/data_aishell3.tgz
|
||
```
|
||
```shell
|
||
# Aishell
|
||
|
||
wget https://openslr.elda.org/resources/33/data_aishell.tgz
|
||
```
|
||
#### 2.1.2 数据批量解压
|
||
```shell
|
||
# 该指令为解压当前目录下的所有压缩文件
|
||
|
||
for gz in *.gz; do tar -zxvf $gz; done
|
||
```
|
||
### 2.2 encoder模型训练
|
||
#### 2.2.1 数据预处理:
|
||
需要先在`pre.py `头部加入:
|
||
```python
|
||
import torch
|
||
torch.multiprocessing.set_start_method('spawn', force=True)
|
||
```
|
||
使用以下指令对数据预处理:
|
||
```shell
|
||
python pre.py <datasets_root> \
|
||
-d <datasets_name>
|
||
```
|
||
其中`<datasets_root>`为原数据集路径,`<datasets_name>` 为数据集名称。
|
||
|
||
支持 `librispeech_other`,`voxceleb1`,`aidatatang_200zh`,使用逗号分割处理多数据集。
|
||
|
||
### 2.2.2 encoder模型训练:
|
||
超参数文件路径:`models/encoder/hparams.py`
|
||
```shell
|
||
python encoder_train.py <name> \
|
||
<datasets_root>/SV2TTS/encoder
|
||
```
|
||
其中 `<name>` 是训练产生文件的名称,可自行修改。
|
||
|
||
其中 `<datasets_root>` 是经过 `Step 2.1.1` 处理过后的数据集路径。
|
||
#### 2.2.3 开启encoder模型训练数据可视化(可选)
|
||
```shell
|
||
visdom
|
||
```
|
||
|
||
### 2.3 synthesizer模型训练
|
||
#### 2.3.1 数据预处理:
|
||
```shell
|
||
python pre.py <datasets_root> \
|
||
-d <datasets_name> \
|
||
-o <datasets_path> \
|
||
-n <number>
|
||
```
|
||
`<datasets_root>` 为原数据集路径,当你的`aidatatang_200zh`路径为`/data/aidatatang_200zh/corpus/train`时,`<datasets_root>` 为 `/data/`。
|
||
|
||
`<datasets_name>` 为数据集名称。
|
||
|
||
`<datasets_path>` 为数据集处理后的保存路径。
|
||
|
||
`<number>` 为数据集处理时进程数,根据CPU情况调整大小。
|
||
|
||
#### 2.3.2 新增数据预处理:
|
||
```shell
|
||
python pre.py <datasets_root> \
|
||
-d <datasets_name> \
|
||
-o <datasets_path> \
|
||
-n <number> \
|
||
-s
|
||
```
|
||
当新增数据集时,应加 `-s` 选择数据拼接,不加则为覆盖。
|
||
#### 2.3.3 synthesizer模型训练:
|
||
超参数文件路径:`models/synthesizer/hparams.py`,需将`MockingBird/control/cli/synthesizer_train.py`移成`MockingBird/synthesizer_train.py`结构。
|
||
```shell
|
||
python synthesizer_train.py <name> <datasets_path> \
|
||
-m <out_dir>
|
||
```
|
||
其中 `<name>` 是训练产生文件的名称,可自行修改。
|
||
|
||
其中 `<datasets_path>` 是经过 `Step 2.2.1` 处理过后的数据集路径。
|
||
|
||
其中 `<out_dir> `为训练时所有数据的保存路径。
|
||
|
||
### 2.4 vocoder模型训练
|
||
vocoder模型对生成效果影响不大,已预置3款。
|
||
#### 2.4.1 数据预处理
|
||
```shell
|
||
python vocoder_preprocess.py <datasets_root> \
|
||
-m <synthesizer_model_path>
|
||
```
|
||
|
||
其中`<datasets_root>`为你数据集路径。
|
||
|
||
其中 `<synthesizer_model_path>`为synthesizer模型地址。
|
||
|
||
#### 2.4.2 wavernn声码器训练:
|
||
```
|
||
python vocoder_train.py <name> <datasets_root>
|
||
```
|
||
#### 2.4.3 hifigan声码器训练:
|
||
```
|
||
python vocoder_train.py <name> <datasets_root> hifigan
|
||
```
|
||
#### 2.4.4 fregan声码器训练:
|
||
```
|
||
python vocoder_train.py <name> <datasets_root> \
|
||
--config config.json fregan
|
||
```
|
||
将GAN声码器的训练切换为多GPU模式:修改`GAN`文件夹下`.json`文件中的`num_gpus`参数。
|
||
|
||
|
||
|
||
|
||
|
||
## 3.致谢
|
||
### 3.1 项目致谢
|
||
该库一开始从仅支持英语的[Real-Time-Voice-Cloning](https://github.com/CorentinJ/Real-Time-Voice-Cloning) 分叉出来的,鸣谢作者。
|
||
### 3.2 论文致谢
|
||
| URL | Designation | 标题 | 实现源码 |
|
||
| --- | ----------- | ----- | --------------------- |
|
||
| [1803.09017](https://arxiv.org/abs/1803.09017) | GlobalStyleToken (synthesizer)| Style Tokens: Unsupervised Style Modeling, Control and Transfer in End-to-End Speech Synthesis | 本代码库 |
|
||
| [2010.05646](https://arxiv.org/abs/2010.05646) | HiFi-GAN (vocoder)| Generative Adversarial Networks for Efficient and High Fidelity Speech Synthesis | 本代码库 |
|
||
| [2106.02297](https://arxiv.org/abs/2106.02297) | Fre-GAN (vocoder)| Fre-GAN: Adversarial Frequency-consistent Audio Synthesis | 本代码库 |
|
||
|[**1806.04558**](https://arxiv.org/pdf/1806.04558.pdf) | SV2TTS | Transfer Learning from Speaker Verification to Multispeaker Text-To-Speech Synthesis | 本代码库 |
|
||
|[1802.08435](https://arxiv.org/pdf/1802.08435.pdf) | WaveRNN (vocoder) | Efficient Neural Audio Synthesis | [fatchord/WaveRNN](https://github.com/fatchord/WaveRNN) |
|
||
|[1703.10135](https://arxiv.org/pdf/1703.10135.pdf) | Tacotron (synthesizer) | Tacotron: Towards End-to-End Speech Synthesis | [fatchord/WaveRNN](https://github.com/fatchord/WaveRNN)
|
||
|[1710.10467](https://arxiv.org/pdf/1710.10467.pdf) | GE2E (encoder)| Generalized End-To-End Loss for Speaker Verification | 本代码库 |
|
||
|
||
### 3.3 开发者致谢
|
||
|
||
作为AI领域的从业者,我们不仅乐于开发一些具有里程碑意义的算法项目,同时也乐于分享项目以及开发过程中收获的喜悦。
|
||
|
||
因此,你们的使用是对我们项目的最大认可。同时当你们在项目使用中遇到一些问题时,欢迎你们随时在issue上留言。你们的指正这对于项目的后续优化具有十分重大的的意义。
|
||
|
||
为了表示感谢,我们将在本项目中留下各位开发者信息以及相对应的贡献。
|
||
|
||
- ------------------------------------------------ 开 发 者 贡 献 内 容 ---------------------------------------------------------------------------------
|
||
|